Predictive maintenance in the food industry
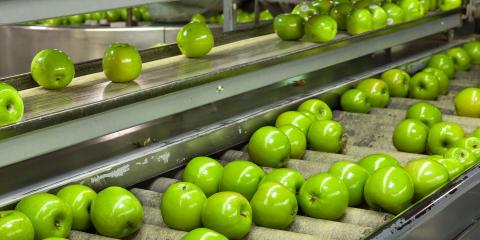
Context
The manufacturing industry is realising that, in many cases, its traditional maintenance strategy - preventive maintenance - is no longer sufficient. Switching to a predictive maintenance strategy reduces operational and maintenance costs.
This new strategy relies on condition monitoring using sensors, data collection and analysis tools. However, the initial investment cost of these technologies can delay the adoption of a predictive maintenance strategy.
It is in this context that a food company conctacted us after some unplanned production stoppages. Asking if we could identify the critical component in the production line? And set up a cost-effective condition monitoring system to better track that vulnerable component?
Our solution
After a thorough analysis of maintenance and MES data, we were able to pinpoint bearing faults in certain extrusion motors as a likely cause of unplanned production interruptions.
To confirm this and enable predictive analysis, we chose vibration-based monitoring of a number of motors. By combining low-cost MEMS-based accelerometer sensors with smart algorithms, this could be done in a cost-effective way.
After selection and benchmarking, we installed these sensors with the necessary filters and data channels on four electric motors in different parts of the production line. An application developed by Flanders Make ran on a central server where a 3-second vibration signal was stored and processed every half hour. In total, we thus collected more than 500 days of valuable data from each motor.
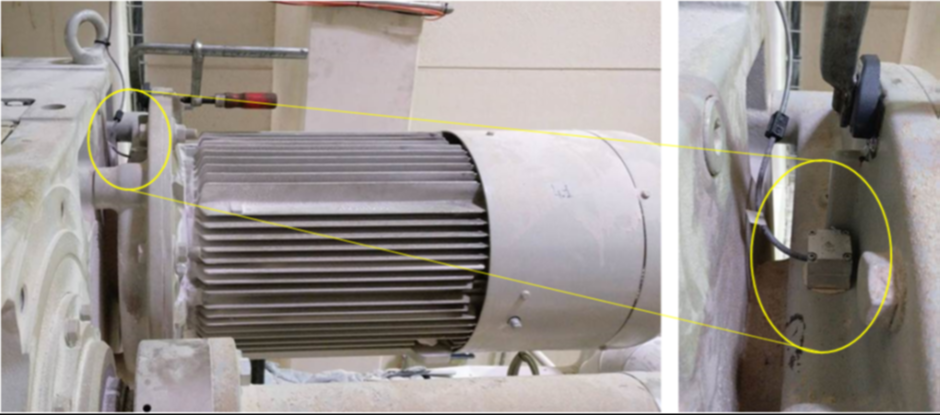
This study confirms the vulnerability of electric motors in the extrusion line. The harsh environmental conditions with large temperature variations and humidity have a clear impact on their lifetime. If, despite periodic maintenance, failure then occurs, the production line abruptly stops and a repair is required followed by a restart procedure of the production line.
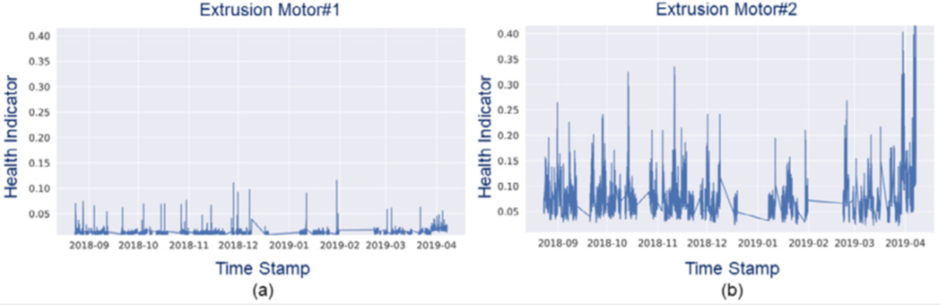
From the raw vibration signals of each motor, our specialists also calculated several bearing health indicators. The evolution of these in one of the extrusion monitors showed a clear negative trend and indicated bearing degradation in this motor.
A maintenance service company was called in to double-check our findings. After confirmation, this then led to the urgent replacement of the failing motor. We were thus able to avoid another abrupt production stop - with accompanying production loss - thanks to a few low-cost sensors combined with the right algorithms.
In the next phase, a roll-out of this kind of condition monitoring to the entire production line is planned.